White paper: Direct indexing transaction costs
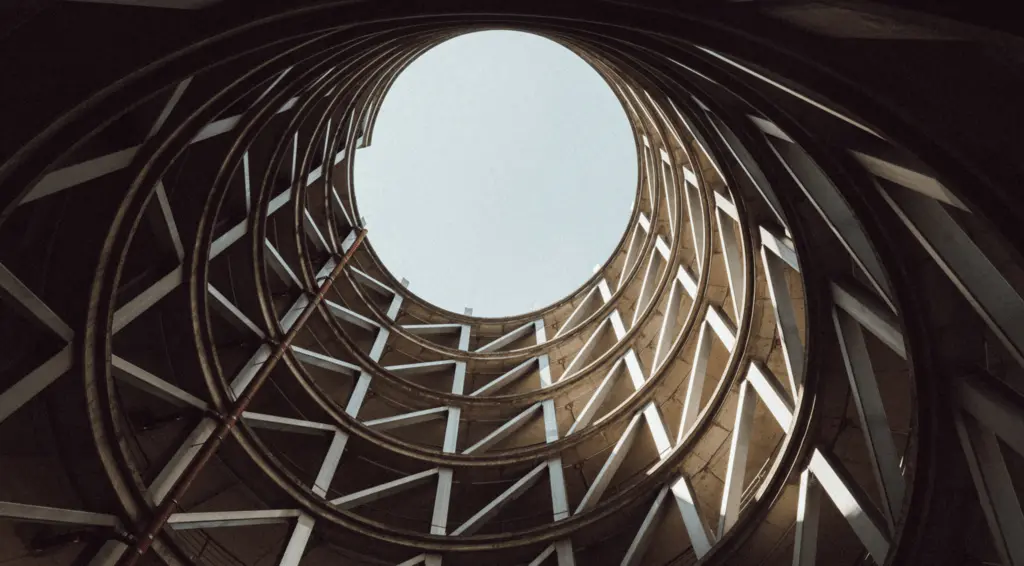
Direct indexing into a large index, such as the S&P 500, involves the automated allocation of several hundred stock positions and frequent trading to harvest tax losses. Like any activity in public markets, each of these transactions will incur transaction costs for the investor. These transaction costs include trading fees as well as costs resulting from the bid-ask spread when executing market orders in an auction market.
In this report we attempt to provide answers to investors interested in how these costs might affect the overall performance of their portfolio when utilizing direct indexing.
Summary of Results
In our analysis, both historical backtest data and recorded trading data indicate that transaction costs resulting from bid-ask spreads are approximately 2 bps (Basis Points, i.e. 0.02%) of trading volume. This finding suggests that these trading costs sum to less than 0.5% of the harvested capital losses on average. Additionally, the trading fees assessed by regulators and passed on to customers add approximately 1.7 bps of trading volume for a small ($50K) account but drop precipitously as the account size grows, e.g. down to 0.12 bps for a $1M account.
Altogether, by estimating trading volumes and harvested tax losses for direct indexing in simulation, we find that that expected transaction costs range between 0.5% to 1% of the harvested tax losses.
Impact of Bid-Ask Spreads
Analysis of historical National Best Bid and Offer (NBBO) data for S&P 500 index constituents over the past 6 years yields a number of interesting observations. First, the magnitude of the bid-ask spreads change significantly, but consistently, throughout a given trading day with spreads typically reducing by factor of approximately 4x from market open to market close. Second, we find that the spreads on smaller market cap stocks are slightly higher than the large caps but not considerably so. Overall, if care is taken to trade toward the end of the trading day, trading costs resulting from bid-ask spreads can be estimated at around 2 bps of the total transaction volume when averaging with respect to index weight.
However, a fixed 2 bps cost per transaction may not fully reflect the NBBO of the specific stocks being traded on a specific date by the direct indexing algorithm. For example, many tax-loss-harvesting opportunities occur during market downturns perhaps the bid-ask spreads are wider at these times. To better approximate costs on a per-stock/per-date level, additional simulations were conducted and analyzed.
Over a set of simulation runs, we find that the average trading costs as a percentage of trading volumes was 0.019%, with all simulations having costs within a range of 0.021% and 0.017%. This provides further support for the previous 2 bps approximation for bid-ask related transaction costs.
This raises the next question: how closely does the NBBO reflect the actual price at which shares are traded in practice? To approximate the difference between the final transaction price and the expected price from the NBBO (aka slippage) we explored results for 9102 sampled direct indexing trades executed on the Frec platform during November 2023. For these trades, the average difference between the final price and the NBBO price (slippage), as a percentage of the final price, was measured at 0.0084%. This indicates that on average these sampled trades the final execution price was very close and in fact slightly better (for the investor) than the expected price (NBBO). Additionally, we find that the estimated transaction costs from bid-ask spread on these recoded trades averaged 2 bps, again consistent with the above observations.
In summary, from the analysis above we conclude that bid-ask spread related transaction costs for a S&P 500 direct indexing approach are well approximated by a 2 bps factor. Note that this factor is a percentage of the total trading volume not portfolio value, we explore estimates of direct indexing trading volumes below.
Impact of Trading Fees
Frec does not charge trading commissions but does pass on fees mandated by the SEC and FINRA. These fees are generally very small but because the fees are rounded up to the nearest cent, the fee relative to account size can be noteworthy for smaller accounts. Specifically, we find that for a $50K direct index account the trading fees can be approximated as 1.7 bps of trading volume while a $1M account can expect trading fees at the 0.12 bps mark.
Costs vs. Benefits
Fortunately for the investor looking to contrast the tax loss harvesting (TLH) benefits with the transaction costs, both of these quantities scale with the total trading volume. In order to develop an understanding of the typical trading volumes we conducted a larger set of 10-year historical backtesting simulations on overlapping periods over a 20 year time span (2003-12-17 to 2023-07-25).
These simulations do not reference the NBBO data directly (as it’s not available) and instead are intended to provide data on total losses harvested and trading volumes. These trading volumes are then used to approximate the transaction costs resulting from the bid-ask spread as well as trading fees using the observations above.

The historical backtesting simulations indicate that on average, direct indexing trading volumes are 9.3x the initial deposit, while harvested tax losses average around 38.9% the initial deposit, over a 10 year period. Therefore, assuming a tax rate of 42.3%1 we calculate a tax savings of 16.1% of the deposit, that is a $8.23K in tax savings on a $50K one-time deposit. Finally, taking 2 bps of the average trading volume of $467K one arrives at the approximate trading costs of around $93 for the same period while 1.7 bps for trading fees result in a $79 cost.
While each investor may make their own conclusions on these findings, we expect that for most investors the tax savings of direct indexing far outweigh the costs associated with increased trading activity.
Bid-Ask Spread Cost Methodology
For larger trading actions, such as those conducted by hedge funds or institutional investors, there are secondary effects of trading that one must consider. For example, the best available bid-ask orders or the order book might lack sufficient volume to fill the market order, leading to a wider-than-anticipated spread as the trader’s order progresses through the order book. Additionally, other market participants such as high-frequency trading algorithms may respond in real-time to large orders in an adversarial manner, increasing costs even further. Transaction costs for these larger transactions have been studied and modeled in some detail for general trading actions [1] and for the direct indexing context specifically [2], [3]. These studies suggest that transaction costs for large-cap US equities can be estimated at around 10 to 12 bps of the trading volume (see [2] and [3] respectively).
However, this report focuses on estimating transactions costs for retail investors employing direct indexing, which are unlikely to trigger such secondary market effects. For these investors, transaction costs are likely more accurately approximated as a function of the the bid-ask spread directly (and trading fees). We believe that currently, and probably for the foreseeable future, this approximation is most relevant for the majority of clients utilizing direct indexing at Frec. Additionally, we explore recorded trading data from the Frec platform to verify this assumption.
Quoted Half-Spread
To begin, we first define a convenient metric for analyzing the bid-ask spread that will be used throughout: the quoted half-spread (QHS) metric, defined as follows for a given time t

Using this quantity it is straightforward to approximate the cost of a given transaction by taking the product of the QHS value with the value of the trade. For example, if the QHS of stock A at the current time is 1% then an investor who purchases $100 worth of stock A, then immediately sells the stock, would have $100 * (100% – 1%)2 = $98.01 cash remaining – i.e. losing 1% of the trade value in each transaction. Throughout this report we use the terms bid-ask spread and QHS interchangeably.
S&P 500 Constituent QHS
The primary dataset used in the following analysis is bid-ask (level 1) data (the NBBO bid-ask quotes) from FirstRate Data, consisting of time series for bid and ask values across numerous stock tickers over the past 10 years. The data can be used to determine the QHS for a given stock for very precise times, though for the purposes of this report we will focus on the QHS averaged over at least 15 minute durations.2
The first question we aim to answer is how the bid-ask spread differs across the S&P 500 constituents, specifically to test the hypothesis that larger constituents have significantly smaller spreads due to increased trading volumes. To get a measure of this we consider a recent month of data, from 2023-10-02 to 2023-11-03, and compute the average QHS for each S&P 500 constituent for each day. Additionally, for reasons we will discuss shortly, the QHS was computed during the final 15 minutes of each trading day 15:45-16:00 ET. Using the resulting 25 data points (each trading day) for each constituent we construct the following figure:

We observe that there is clearly some (negative) correlation between the index weight and the QHS for different constituents. But that generally the bid-ask spreads are not prohibitively bad for the smaller market cap stocks. Instead, we find that the primary factor determining whether constituents have a higher bid-ask spreads seems to be the share price. For instance, tickers such as NVR (~$6k/share), BKNG (~$3k/share), and AZO (~$2.6K/share) are amongst the outliers exhibiting the largest spreads.
Intra-day Bid-Ask Spreads
Another important aspect of bid-ask spreads is how they typically change throughout the course of the trading day. In order to refine the QHS for each constituent down to a single number we consider an index-weighted QHS factor, which is defined as the sum of all the constituent QHS values multiplied by the corresponding S&P 500 index weight; or the index-weighted QHS if you will. This quantity represents the transaction costs one would expect to incur when purchasing the entire S&P 500 index in the appropriate allocations. In the following figure statistics for the index-weighted QHS are shown for each 15 minute period, over the same 2023-10-02 to 2023-11-03 period used above.

In the previous section we consider the QHS values averaged over the final 15 minutes of each trading day, the reasons for this choice are likely evident to the reader at this point. For this dataset the reduction in the QHS for the final 15 minutes of the trading day compared to the first 15 minutes is approximately 4x. This phenomenon is well known in the industry, but such observations clearly indicate the importance of trading toward the end of the day for automated trading applications such as direct indexing.
Market Volatility
Another important consideration for direct indexing applications is how the bid-ask spread changes during periods of market uncertainty, particularly downturns. This is of utmost importance because it is during these periods that tax loss opportunities surface and, therefore, when tax loss harvesting algorithms typically increase trading volume. To get a better understanding of the bid-ask spreads during such periods we consider the index-weighted QHS for each (full) trading day in the year 2020, where the Covid-19 pandemic caused considerable market volatility.

In the above figure we observe that the index-weighted QHS changes significantly during late February and throughout March as markets reacted early in the Covid-19 pandemic. Additionally, we see that while the QHS values reduced from their peaks in late April they remained elevated from the January baseline throughout the remainder of the year.
Summarizing the findings in this section, an ordinary retail investor can reasonably expect that trading costs resulting from the bid-ask spread can likely be kept below 2 bps, if one is careful to avoid trading near market open. In the following section we consider the impact of the bid-ask spreads over multi-year periods (including the 2020 period shown above) using direct indexing simulations.
Historical Backtesting
One limitation of the the index-weighted QHS approximations above is that they do not consider the specific stocks bought and sold by a direct indexing algorithm. Perhaps, for example, it is possible that bid-ask spreads are higher exactly when tax loss harvesting opportunities arise and the stocks are traded.
In order to attain a more granular approximation of the trading costs we make use of historical backtesting simulations, as described in the Direct Indexing White Paper. The direct index simulations assume a single one-time deposit of $50,000 and no additional reinvestment of tax savings3. The optimization used for the simulations is the same as in the original white paper with the exception that the optimization objective has been updated to add a transaction cost penalty, parameterized by γ. Specifically, we have

where γ is set to 0.002 (20 basis points).4 This term moderates trading activity by effectively subtracting the transaction costs from harvested losses in the optimization, i.e. to ensure the trade is worthwhile.
To approximate the trading costs using this simulation data we are able to take each individual trade and multiply by the estimated QHS for that stock on that day. Here we use the average QHS values for the last 15 minutes of the trading day.
However, detailed simulations using the NBBO are challenging to conduct, primarily due to data cost and quality issues for the order book data. For this report we elected to use a rather simple dataset but even so the full dataset is approximately 24 TB in size. Additionally, the reliability of data diminishes as one looks further back in time, with an increase in both missing and incorrect information. This is typically due to corporate actions such as mergers and acquisitions, symbol changes, and so on.
In order to balance the data-quality issues and simulation length we elected to consider 5-year simulations, staggered by 3 months, conducted over the period from 2018-01-01 to 2023-07-03 for this analysis. In the figure below the time series of trading volume for a specific example simulation (from 2018-01-01 to 2022-01-03) is shown.

For this simulation a total value of $469K was traded, of the individual trades making up this sum $29.3K worth were removed due to missing NBBO data.5 For the remaining $440K of trading volume the total approximate trading cost (summing over each individual trade) came out to $78.76 or 0.018% (1.8 bps) of the trading volume.
Looking at the entire set of simulation runs, 7 in total, the average trading costs as a percentage of trading volumes were 0.019%, and all simulations exhibited costs within a range of 0.021% and 0.017%. Overall this is consistent with the approximations in the previous section with bid-ask related transaction costs just below the 2 bps mark.
Recorded Trading Data
Above we observe that transaction costs can be approximated at around 2 bps if the trader is able to fill their orders at the NBBO bid and ask. The question remains, however, how often can orders be expected to fill within this range in practice? The difference between the price an investor expects to get (the NBBO) and the price the order actually fills at, is referred to as slippage. Specifically, if the investor receives a better price than expected then the slippage is positive while a worse-than-expected price yields a negative slippage, that is:

To obtain a measure of the realized slippage for a direct indexing platform like Frec’s it is necessary to look at empirical trading data. Frec executes direct indexing trades via separate market orders for each account in a sequential randomized order. Additionally these orders are submitted when bid-ask spreads are near their daily lows (i.e. toward the end of the trading day).
Taking a sampling of 9102 direct index trades executed in November 2023, and the NBBO collected at algorithm run time,6 we observed the following statistics:

The observations from these data are generally favorable, the positive slippage here indicates that on average orders filled at a better-than-expected price – i.e. inside the NBBO spread. The QHS for these trades averaged out to 0.021%, so an Avg. %-Slippage result of 0.0084% implies that the realized QHS for these trades was approximately 0.02% (2 bps). That is to say, these data suggest the final execution prices for direct index trades on Frec very closely match the prices one would expect looking at the NBBO values at order time. Additionally, the average QHS for these orders is consistent with the results obtained from historical data detailed in the preceding sections.
There are however a few caveats to point out, first of all the dataset used in this analysis is small, and considers only dates within a single month — we hope to provide updates on these numbers as we collect more data. Additionally, the Direct Indexing product at Frec is just over a month into launch so trade volumes are yet not very large. As the product matures and account sizes and order sizes grow, it is important for the platform to continuously monitor trading costs and adapt the automated trading algorithms appropriately.
Regulator Trading Fees
Frec does not charge a commission on trades but there are mandatory fees on selling stock put in place by the SEC and FINRA, which are passed on to the customer. The SEC fee is currently $8 per $1M of stock sold, or 0.08 bps while the FINRA TAF fee is $1.45 per 10,000 shares sold. Both fees are rounded up to a minimum of $0.01. This rounding up to the nearest cent has a disproportionate effect on smaller accounts, as illustrated by the following figure:

The above figure is constructed from historical backtesting simulations with different deposit sizes. The trading fees are calculated based for each simulated trade, the sum of these fees are shown as a percentage of the total trading volume for the simulation. Smaller accounts are more affected by the fee than larger accounts because the total number of trades remains fairly constant as we vary the deposit size but the volume (i.e. value) of trades is scaled proportionally. As a result the large majority of trades (99.9% in the $50K deposit setting) are very small but the fee is rounded up to $0.01.
Note that the fee is only charged on sell orders, but we still look at the fee as a function of the total trade volume (buys and sells) to make for a straightforward comparison with the bid-ask related costs.
In summary, we observe that the trading fees are around the same level of impact as the 2 bps observed from bid-ask spreads for smaller accounts (<$50K) but the relative impact drops precipitously as the account size grows.
Long Term Costs vs Benefits
The observations above all indicate that transaction costs can be approximated as a fixed percentage of the trading volume. Armed with this information we can directly compare the tax loss harvesting benefits of direct indexing with the transaction costs on robust simulation data.
In this section, we consider data collected from 10-year simulation runs for the direct indexing algorithm on the S&P 500 index for staggered 3-month periods over the date range 2003-12-17 to 2023-07-25 (40 simulations). Again, a one-time $50K deposit was used as was the same objective function detailed above.
We first observe that the average trading volume over the 40 simulations was approximately $467K or 9.3x the initial deposit over the 10 year period.

It’s clear that direct indexing involves a considerable amount of trading volume relative to the funds deposited. This observation underscores the significance of accounting for transaction costs when evaluating the feasibility of direct indexing strategies.
Additionally, when averaged across simulation runs the total harvested losses were $19.5K, or 38.9% of the initial deposit. In order to determine the approximate tax savings from this number we can multiply these loses by a fixed tax rate. Here we use the 42.3% tax rate which represents an investor in the 95-98th percentile tax bracket as is described in the “Investor A” profile of Khang et al. [4], though the avid reader may wish to substitute in a value they find appropriate for their situation.
Additionally, on the transaction cost side, we can utilize the 2 bps estimate for the bid-ask related costs and the 1.7 bps for trading fees from earlier sections. The following figure illustrates the the relevant quantities.

The simulation data indicate that the trade costs resulting from bid-ask spreads represent around 2.1% of the total tax savings for the simulation period and for an assumed tax rate of 42.3%. Also, we note that the impact of the trading fee component would decrease significantly for larger accounts. Overall we conclude that the cost benefit analysis is fairly lopsided in favor of direct indexing as it related to tax savings versus transaction costs, even at tax rates as low as 1% the investor is still able to come out ahead.
Discussion
The different analysis conducted in this report consistently indicate that transaction costs resulting from bid-ask spreads are approximately 2 bps. Additionally, the regulator trading fees passed on to the customer can be estimated at around 2 bps for small accounts but far less for larger accounts.
These results seem to indicate transaction costs are lower than the approximation appearing in the literature, e.g. the 10 bps detailed in Liberman et al. [2]. Though, if one were to use these more conservative approximations, the transaction costs are stil insignificant when compared to the potential tax savings for direct indexing for most investors.
Finally we note that additional optimizations could be applied to the direct indexing optimization to further reduce transaction costs. For example, the transaction cost term used in the optimization objective for direct indexing used a constant value of 5 bps for all stocks. However, the data suggest that actual costs vary greatly across the different S&P 500 constituents. A similar observation can be made over time, as the bid-ask spreads change significantly during periods of higher market volatility. Therefore, setting the transaction cost multiplier in the optimization to reflect the real-time NBBO quote for each constituent may yield improved performance.
References
[1] Almgren, R., C. Thum, E. Hauptman, and H. Li. 2005. “Direct Estimation of Equity Market Impact.” Working paper. Available at Computer and Information Science, University of Pennsylvania: https:// http://www.cis.upenn.edu/~mkearns/finread/costestim.pdf.
[2] Liberman, Joseph and Krasner, Stanley and Sosner, Nathan and Maia de Freitas, Pedro Paulo, Beyond Direct Indexing: Dynamic Direct Long-Short Investing (May 3, 2023). The Journal of Beta Investment Strategies, Direct Indexing Special Issue 2023, 14 (3): 10-41; DOI: 10.3905/jbis.2023.1.045
[3] Goldberg, L. R., T. Cai, and P. Hand. 2022a. “Tax Rate Arbitrage: Realization of Long-Term Gains to Enable Short-Term Loss Harvesting.” Journal of Investment Management 20 (3): 12–32.
[4] Khang, Kevin and Cummings, Alan and Paradise, Thomas, and O’Connor, Brennan, Personalized Indexing: A Portfolio Construction Plan, (March 2022), https://corporate.vanguard.com/content/dam/corp/research/pdf/personalized_indexing_a_portfolio_construction_plan.pdf
This white paper describes implementation and performance details of a Direct Indexing approach similar to that used on the Frec platform at the time of writing (11/24/2023) details may differ from the implementation used in the product now and in future. A list of notable corrections follow. 12/4/2023: Transaction cost multiplier was incorrectly stated as 5 bps, 20 bps was used in simulation, the text has been changed to reflect this.
This white paper is for information purposes only and is not intended as tax advice. The data provided above, except trading data discussed in the Recorded Trading Data section, is based on results from historical simulations conducted with Frec’s Direct Indexing Model tracking the S&P 500 index. The results are hypothetical, do not reflect actual investment results, and are not a guarantee of future results. Past trade execution performance is also not a guarantee of future results.
The S&P 500® index is a product of S&P Dow Jones Indices LLC or its affiliates (“SPDJI”), and has been licensed for use by Frec Markets, Inc. S&P®, S&P 500®, US 500 and The 500 are trademarks of Standard & Poor’s Financial Services LLC (“S&P”); and these trademarks have been licensed for use by SPDJI and sublicensed for certain purposes by Frec Markets, Inc. Frec’s direct indexing strategy is not sponsored, endorsed, sold or promoted by SPDJI, Dow Jones, S&P, their respective affiliates and none of such parties make any representation regarding the advisability of investing in such product(s) nor do they have any liability for any errors, omissions, or interruptions of the S&P 500® index.